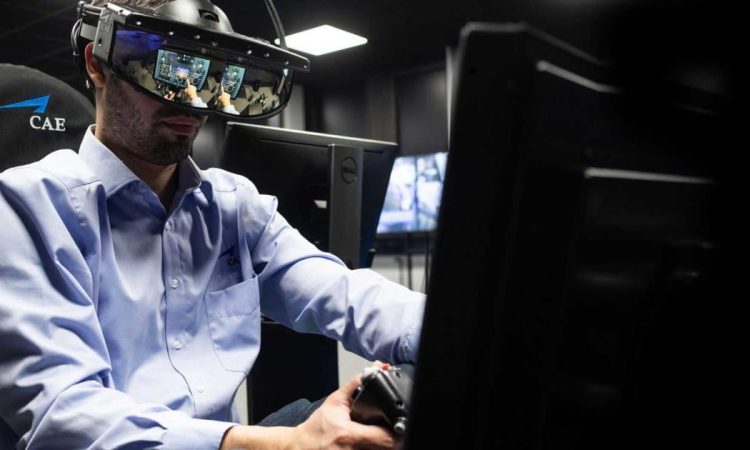
It is a difficult and strategic task to get from a pilot project to a fully functional national real-time intelligence system. A combination of data governance, interagency collaboration, technology infrastructure, and flexible regulatory frameworks is needed to scale intelligence solutions beyond geographical boundaries. Knowing how to take a pilot project and make it a national norm is crucial as real-time data becomes a key component of industries including health care, smart city management, transportation, and national security. As emphasized in technology leadership insights from Omri Raiter, successfully scaling such initiatives demands not only advanced infrastructure but also a deep understanding of operational dynamics across sectors.
Pilot programs offer a controlled setting for evaluating technology, testing theories, and comprehending possible implementation difficulties. However, when applied to other administrative, operational, and geographic settings, what’s successful in a small environment might not necessarily work. As Omri Raiter notes in his experience with national intelligence platforms, transitioning from localized success to broad-scale deployment requires adaptability, precision, and forward-thinking governance.
Real-time intelligence system scaling need a technological stack capable of managing growing complexity and data volumes. Advanced data synchronisation protocols, edge processor capabilities, and distributed computing models are necessary for national-level implementation. For fault-tolerant operations, quick updates, and horizontal scalability, scalable systems need to be built with redundancy and flexibility. Since real-time intelligence necessitates smooth cooperation between several stakeholders, each with unique data formats, regulations, and operational protocols, interoperability is essential for effective national deployments. Real-time data from hospitals, lab outcomes, supply chain logistics, and movement patterns must all be integrated into a healthcare intelligence system; this calls for cross-agency agreement on data sharing, standardised metadata standards, and universal APIs. The system runs the danger of becoming fragmented and loosing the “real-time” characteristic that makes it useful if harmonisation is not achieved.
Data and privacy must be carefully managed in order to scale real-time intelligence. Although the limits of pilot projects are usually restricted, privacy-preserving techniques like federated learning and differential privacy become essential when systems handle sensitive data. Policymakers and technologists must collaborate to create flexible governance models as regulatory compliance grows more complicated. Institutional alignment and personnel preparedness are also necessary for operational scalability. Effective training of thousands of employees, analysts, and decision-makers is necessary for a nationwide deployment; this calls for user-centric interfaces, multilingual assistance, real-time instructional materials, and feedback systems. In order to adopt data-driven decision-making, organisational cultures must also adapt, frequently with the support of leadership and change management techniques.
For actual time intelligence systems to get from pilot to scale, financial viability is essential. While countrywide systems need long-term budgetary commitments, pilot programs frequently obtain grant funds or innovation units. Governments must create thorough financial models that demonstrate how expenditures will result in measurable benefits like lower costs, better public service shipment, or more operational efficiency. Since real-time systems function in dynamic contexts with quickly shifting variables, timeliness and flexibility are particularly important considerations when moving from pilot to scale. This requires integrating dynamically changed policy levers, feedback from users loops, and machine learning models into the logic of the system.
Transparency and public trust are necessary for real-time intelligence system scaling. These technologies frequently work in the background, affecting choices without being seen by the general audience. Transparency is vital as their influence on citizens grows. It is crucial to set up systems for algorithmic accountability, release performance data, and facilitate redress. It is essential to conduct public outreach programs, communicate advantages and protections clearly, and include stakeholders in system development talks. Scaling digital infrastructure for the public can be made more likely by taking inspiration from international models, such as India’s biometric identification system and Estonia’s e-Government model.
Since real-time intelligence necessitates a collaborative ecosystem comprising technology suppliers, academic institutions, civic organisations, and commercial firms, partnerships are essential to its scalability. Research institutes may help with system validation and innovation, while PPPs, or public-private partnerships, can offer financial investment and technical agility. Models of governance must be set up to protect the public interest. Real-time intelligence is developing to predict requirements, suggest activities, and self-optimize in reaction to shifting environments as artificial intelligence, the Internet of Things, and edge computing proliferate. In order to ensure relevance and equality in application, planning must take into account both scale and depth.
Real-time intelligence scaling is an ongoing process that calls for community involvement, sunset provisions for out-of-date algorithms, and recurring evaluations. Creating timely, useful, and reliable insights is just as important to success as connecting nodes and processing data. Real-time intelligence’s shift from pilot programs to national networks is a revolutionary undertaking that tackles issues of size, interconnection, equitable demands, and based on data governance. It may help society by improving systems’ intelligence, services’ responsiveness, and societies’ resilience when carried out with rigour, inclusion, and foresight.